New Product Forecasting - Line Extension #2

By Roei Aviram, CEO at Intelichain
As mentioned in our previous article, “Line Extension” is the most prominent NPI category, with about 29% of the total global product launches world-wide. In this type of NPI the company plans to extend its established product brand name with a new product/s in the product category. The company aims to keep you excited and interested in the brand by creating a new product in one of its existing categories targeting a specific customer group. Typical extensions might be in a new flavor, scent, formula, color, size, or style of a product in a current product line. Good examples might be: Colgate extended the classic toothpaste into additional SKUs such as: Colgate Max White, Colgate Max Fresh, Colgate Max Fresh with Whitening, Coca-Cola introduced a cherry flavor, Hellmann’s Mayonnaise with Olive Oil, etc.
In order to accurately forecast this type of NPI, the best practice approach would first suggest you to be based on internal datathat exists for a similar “Look-Alike” products. The extended and the new product should have a highly correlated behavioral patterns since the new product relies on the assumption both products are focusing on the same target audience and preferences.This similarity is called Look Alike modeling, a ML model that calculates the degree of similarity based on considered attributes. This model gained popularity due to its simplicity and availability and could be best approach to tackle this business problem, especially for retailers with huge amount of SKUs in their product portfolio.
Having that said, to really maximize accuracy, pure statistics won’t be enough. The leading practice suggests you incorporate external datasuch as market research, executive knowledge, expert opinion, trusted customers interviews, suppliers, and other sales partners such as dealers or distributors so we get their take on how the product will do, and any other qualitative data that might enrich our statistical forecast.
In Line Extension there is another aspect which we would need to pay attention to – the Cannibalism effect. Obviously, a company extends a product line to address other customers’ needs that are not fully fulfilled in the existing product, and of course to gain higher market share at the expense of your competitors. Little do they know that in most cases, launching extended product actually cannibalize your own existing product or products. Therefore, launching products from Line Extension category should result in evaluating the impact of the entire product line and associated products, to adjust weights on the most impacted products trying to rely on similar launches and constantly adjust assumptions with time.
4 Possible cannibalism effect:
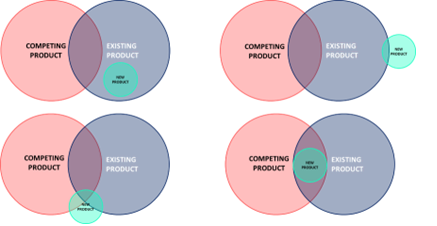
In addition to Canibalism analysis, another aspect you would want to look at, especially for seasonal products, is the time in the year you launched. To forecast seasonality, we would need a history of not less than 2 years (where 3 years is the best-practice).
However, when forecasting a new product, we don’t have much history to understand this a seasonal item we are trying to forecast. Therefore, we would use the historical sales data of the most similar product to capture seasonality and enrich our forecast with assumptions. An annual demand forecast, even a qualitative one, would normally be somewhat accurate so if we could extract the seasonal factors, we can capture a much more more realistic demand pattern.
Example of seasonal factors:

To the seasonal indexes we will add, or multiple, the trend component to make sure our forecast continues the overall trend of the market. Once put together, we will enrich our forecast with qualitative assumptions and generate a consensus forecast for this NPI item. Every month we will review our NPI forecast accuracy in the S&OP Demand Review Meeting adjust our assumptions if needed.
Another great approach that would help you optimize your NPI forecast accuracy is a Top-Down (TD) approach. Top-Down approach captures the family/ category trend and eliminates the SKU variability, especially when you don’t have enough history. The TD approach would help you understand the stable trend and correct adjustments according to the size of the past demand for each SKU.
To really understand the written above, let’s see an example that would look (more or less) the same for both approaches.
Company XYZ is going to launch a new product that would be a line extension for a popular peanut-butter ice-cream flavor. The company uses historical sales data of the past 4 years, from January 2018 to December2021.
The key question we would like to address is what would the demand pattern of the year 2022 be?

In case this in not a seasonal product the best approach would be to apply a simple exponential smoothing, where this model uses weighted averages of past demand observations to forecast new demand values. Since in NPI items responsiveness is a key factor, it is recommended to apply a high value for alpha, say 0.7-0.9, to capture changes and adapt quickly, rather than a low alpha which would smooth out your forecast and wont catch sharp market changes.
However, if it’s a seasonal product, as mentioned before we will extract seasonal factors and apply it on our total yearly estimate.
In the below example we can demonstrate the historical sales data of a similar product and the question we would raise be: what would the forecast for the next 12 months be?

In case we failed to understand a seasonal adjustment must be made, and in every typical forecasting system (since we have no past data to determine our seasonal factors) the system will most likely try to smooth out the demand using exponential smoothing. Therefore, the forecast most likely would look somewhat like this:
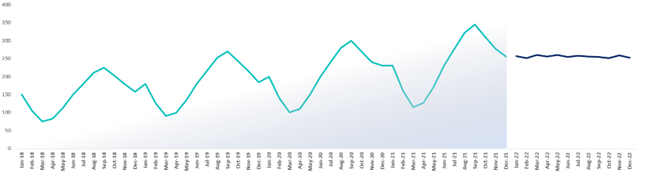
However, we obviously don’t really think this is what the future demand would actually look like… It will be safe to say the year 2022 most likely would look somewhat look like the below image:

This is where demand planning best-practice comes-in!
So lets recap, any external information from market research, sales force team, experts’ opinion, market survey and so forth might be very much valuable to enrich the demand forecast. In addition to that, a demand planning software with advanced analytical capabilities can make a serious difference, even without external data.
Using AI and ML capabilities can easily and quickly detect similar types of products which have a similar demand pattern, based on clustering analysis which takes into consideration multiple product’s attributes such as: product type, season of introduction, price, demographics, size, color and much more. Once detected, the system could extract effective and similar seasonal factors and to apply that launch profile on your NPI forecast.
The combination of Demand Planning best-practice with skilled demand planners, marketing planners and sales team together with analytical platform can make really make a significant impact to your business results. The power of data is incredible and can improve your forecast accuracy dramatically.